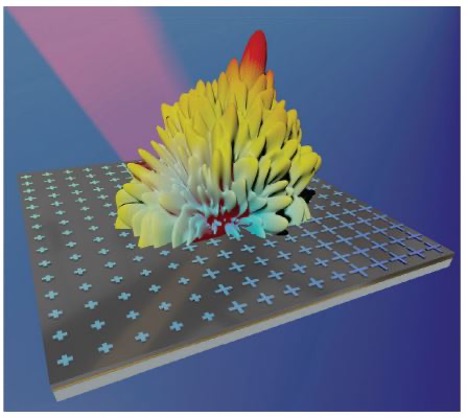
Metasurfaces are a revolutionary development in the world of optics. These are extremely sophisticated, customizable surfaces that have been tailored to reflect, absorb, or transmit light based on the designer’s needs. The complex structures on the material’s surface are of a sub-wavelength scale compared to the wavelength of interest. These structures act as “mini transmitters and receivers” that will reflect, absorb, or transmit select regions of the electromagnetic spectrum. This novel invention is promising for stealth applications, microscope and telescope technology, and more.
There are quite a number of variables to consider when designing a metamaterial: the desired wavelength range, absolute reflectance, specular versus diffuse reflectance, and more. On top of that, the individual surface features correspond to a particular wavelength, meaning that eliciting a broadband response proves to be challenging. As such, machine learning (ML), which uses algorithms to process and analyze datasets to produce a model capable of inferences and predictions, has been integrated into metasurface design. Even still, the necessity for many large metamaterial datasets, as well as the intricate and large-scale geometry of the design, limits the speed and accuracy of using ML in metasurface design.
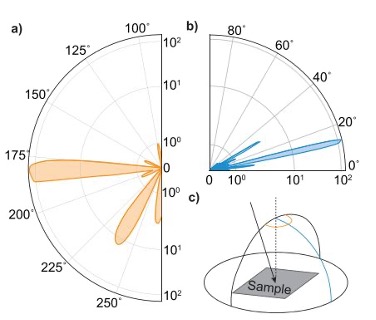
Figure 1: BRDF Data collected from the SOC-210 BDR with an incident beam at a wavelength of 10 micrometers and fixed position (θi = 13°, Φi = 0°). a) Forward-scattered half of the principal ring (θR = 13°, ΦR = 90 – 270°). b) Forward-scattered half of the in-plane slice (θR = 0 – 90°, ΦR = 180°), BRDF intensity is in log scale. c) A diagram displaying the principle ring (orange) and in-plane slice (blue) corresponding to Figures 1a and 1b.
To minimize the time and maximize the accuracy of metasurface design, a team from Duke University combined computational electromagnetic software with analytical models to construct optical datasets. Through ML, the optical datasets were then processed and analyzed to develop a customized metasurface diffuser design. With this method, Rozman et al. tackled the computational limitations that came with constructing metasurface datasets and fabricated material with a diffuse scattering in the mid-IR.
To test the efficacy of their novel method, they used the SOC-210 bidirectional reflectometer (BDR, 0.40 – 14 micrometers) and the SOC-100 hemispherical-directional reflectometer (HDR, 2.0 – 200 micrometers). The SOC-210 BDR was used to characterize the bi-directional reflectance distribution function (BRDF) of the metasurface at 10 micrometers. The BRDF values were measured across the principal ring (Figure 1a) and the in-plane slice (Figure 1b). The measured angles are visually represented in Figure 1c. In Figures 1a and 1b, the large lobes at 180° (Figure 1a) and 13° (Figure 1b) are indicative of a specular lobe centered on the point where the two ring and slice converge (Figure 1c). The other observed lobes are ordered by diffusely scattered light controlled by the nature of the metasurface material. The BRDF measurement allowed the team at Duke University to compare the empirical results with the theoretical expectations.
The SOC-100 HDR measurements in Figure 2 demonstrate that the diffuse reflectance was about 30% higher than the specular reflectance at 9.647 microns. Ultimately, the specular reflectance of the metasurface diffuser decreased within 9.09 – 12.5 micrometers independent of the incident angle or polarization in comparison to a sample of the same material with no surface design (Rozman et. al.).
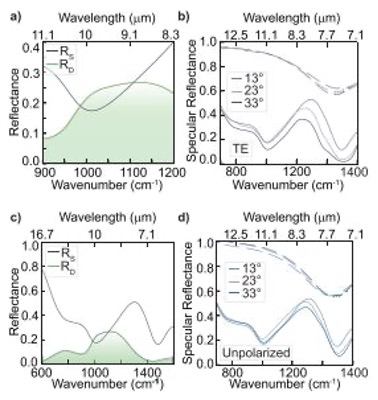
Figure 2: Metasurface reflectance data collected using the SOC-100 HDR. The diffuse reflectance is in green, and the specular reflectance is in gray for Figures 2a and 2c. In Figures 2b and 2d, the specular reflectance is displayed as a solid line while diffuse reactance is shown as a dashed line, with b) displaying polarized light and d) displaying unpolarized light.
This experiment proved the efficacy of combining optical models with ML practices to minimize computational time and increase the accuracy of metasurface design. The accelerated learning from ML models combined with sophisticated optical tooling opens a new field of metasurface materials for application-tailored optical design controlling total reflectance and angle-dependent optical signal.